This project was conducted by Dr. Carrie Demmans Epp, Dr. Carla Hudson Kam, Dr. Jenny Thomson, Julia Rivard, Neesha Desai, and Emilia Hidalgo (Undergraduate Student).
In literacy education, students can struggle to remain motivated to work on skills that are difficult for them. Adding game elements to literacy education through e-learning tools can help to keep students engaged with the material. However, it is important to ensure that the material used is appropriate as content that is too hard or too easy could detract from the quality of the education and student engagement. This project developed methods to evaluate questions in a literacy-focused educational game. This method uses a hybrid of automated analysis and human inspection to streamline the continual revision and improvement of questions.
We analyzed student performance across grade level and literacy skill type to identify both specific questions and broader areas that need improvement. We applied our methods to multiple sets of questions and achieved similar results. The data we analyzed were from just under 5,000 students in grades 1 through 8.
We used clustering to identify questions that may be less appropriate because students answered them either consistently correctly or incorrectly. The questions that were flagged through the clustering process highlighted skill types at particular grade levels with a high number of questions that needed review. The presence of multiple flagged questions within the same skill type and grade was often an indicator of a larger performance trend. Student performance on questions by skill type and grade can be seen in Figure 1.
Our results were shared with the game’s content developers so that they could be used to address potential shortcomings. We communicated the flagged individual questions and areas for improvement back to the company so they could start the process of improving questions by editing the question itself or by recalibrating the question’s difficulty. One such question had very low performance, because it relied on a word at a higher reading level than the question was intended to evaluate. Since the question was flagged, the content developers were able to identify the source of the problem. They then changed the word to better fit the intended difficulty level.
Our methods were developed using a subset of the questions from the game. We then applied these methods to data collected from a separate subset of questions, where we observed similar results. The use of this approach to identify potentially problematic questions in two datasets demonstrates its potential to enable the continual monitoring and improvement of questions used in educational tools.
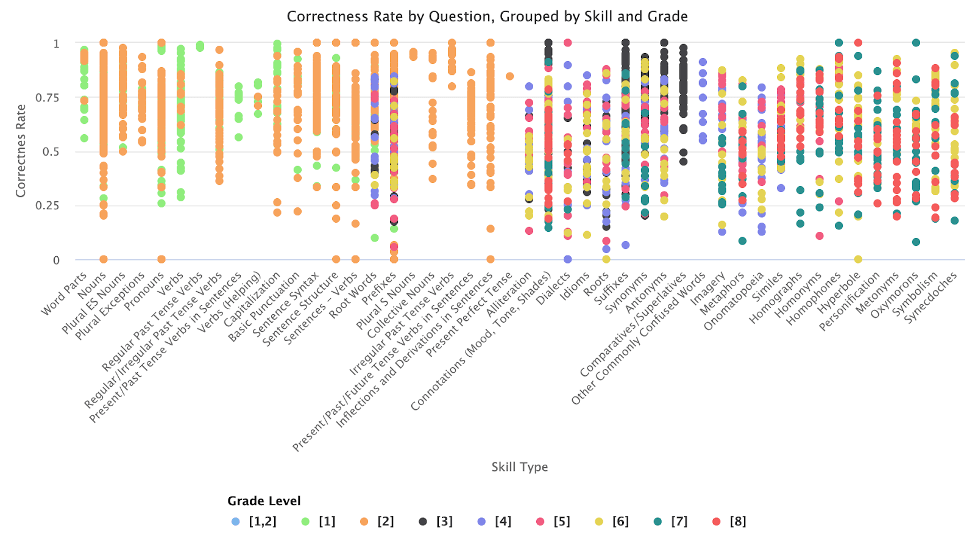